[ad_1]
Keeping up with an industry as fast-moving as AI is a tall order. So until an AI can do it for you, here’s a handy roundup of the last week’s stories in the world of machine learning, along with notable research and experiments we didn’t cover on their own.
In one of the more surprising stories of the past week, Italy’s data protection authority (DPA) blocked OpenAI’s viral AI-powered chatbot, ChatGPT, citing concerns that the tool breaches the European Union’s General Data Protection Regulation. The DPA is reportedly opening an investigation into whether OpenAI unlawfully processed people’s data, as well as over the lack of any system to prevent minors from accessing the tech.
It’s unclear what the outcome might be; OpenAI has 20 days to respond to the order. But the DPA’s move could have significant implications for companies deploying machine learning models not just in Italy, but anywhere within the European Union.
As Natasha notes in her piece about the news, many of OpenAI’s models were trained on data scraped from the Internet, including social networks like Twitter and Reddit. Assuming the same is true of ChatGPT, because the company doesn’t appear to have informed people whose data it has repurposed to train the AI, it might well be running afoul of GDPR across the bloc.
GDPR is but one of the many potential legal hurdles that AI, particularly generative AI (e.g. text- and art-generating AI like ChatGPT), faces. It’s becoming clearer with each mounting challenging that it’ll take time for the dust to settle. But that’s not scaring away VCs, who continue to pour capital into the tech like there’s no tomorrow.
Will those prove to be wise investments, or liabilities? It’s tough to say at present. Rest assured, though, that we’ll report on whatever happens.
Here are the other AI headlines of note from the past few days:
- Ads come to Bing Chat: Microsoft last week said that it’s “exploring” putting ads in the responses given by Bing Chat, its search agent powered by OpenAI’s GPT-4 language model. As Devin notes, while the sponsored responses are clearly labeled as such, it’s a new and potentially more subversive form of advertising that may not be as easily delineated — or ignored. Plus, it could further erode trust in language models, which already make enough factual errors to sow doubt in the veracity of their responses.
- A request for a pause: A letter with more than 1,100 signatories, including Elon Musk, published on Tuesday called on “all AI labs to immediately pause for at least six months the training of AI systems more powerful than GPT-4.” But the circumstances surrounding it turned out to be murkier than one might’ve expected. In the subsequent days, some signatories walked back their positions while reporting revealed that other notable signatories, like Chinese president Xi Jinping, turned out to be fake.
- And a response to the pause request: Prominent AI ethicists point out that worrying about distant, hypothetical issues is dangerous and self-defeating if we don’t address the problems AI is contributing to today.
- Twitter reveals its algorithm: As repeatedly promised by Twitter CEO Elon Musk, Twitter has opened a portion of its source code to public inspection, including the algorithm it uses to recommend tweets in users’ timelines. Interestingly, Twitter appears to rank tweets in part using a neural network continuously trained on tweet interactions to optimize for positive engagement, like likes and replies. But there’s a lot of nuance to it, as the researchers digging into the codebase note.
- Summarizing meetings with AI: Following on the heels of companies like Otter and Zoom, meeting intelligence tool Read has introduced a new feature that trims an hour-long meeting into a two-minute clip, accompanied by important pointers. The company says it’s using large language models — it didn’t specify which ones — combined with video analysis to pick out the most notable parts of the meeting, a useful feature.
More Machine Learnings
At AI enabler Nvidia, Bionemo is an example of their new strategy, where the advance is not so much that it’s new, but that it’s increasingly easy for companies to access. The new version of this biotech platform adds a shiny web UI and improved fine-tuning of a bunch of models.
“A growing portion of pipelines are dealing with heaps of data, amounts we’ve never seen before, hundreds of millions of sequences we have to feed into these models,” said Amgen’s Peter Grandsard, who is leading a research division using AI tech. “We are trying to obtain operational efficiency in research as much as we are in manufacturing. With the acceleration that tech like Nvidia’s provides, what you could have done last year for one project, now you can do five or ten using the same investment in tech.”
This book excerpt by Meredith Broussard over at Wired is worth reading. She was curious about an AI model that had been used in her cancer diagnosis (she’s OK) and found it incredibly fiddly and frustrating to try to take ownership of and understand that data and process. Medical AI processes clearly need to consider the patient more.
Actually nefarious AI applications make for new risks, for instance attempting to influence discourse. We’ve seen what GPT-4 is capable of, but it was an open question whether such a model could create effective persuasive text in a political context. This Stanford study suggests so: When people were exposed to essays arguing a case in issues like gun control and carbon taxes, “AI-generated messages were at least as persuasive as human-generated messages across all topics.” These messages were also perceived as more logical and factual. Will AI-generated text change anyone’s mind? Hard to say, but it seems very likely that people will increasingly put it to use for this kind of agenda.
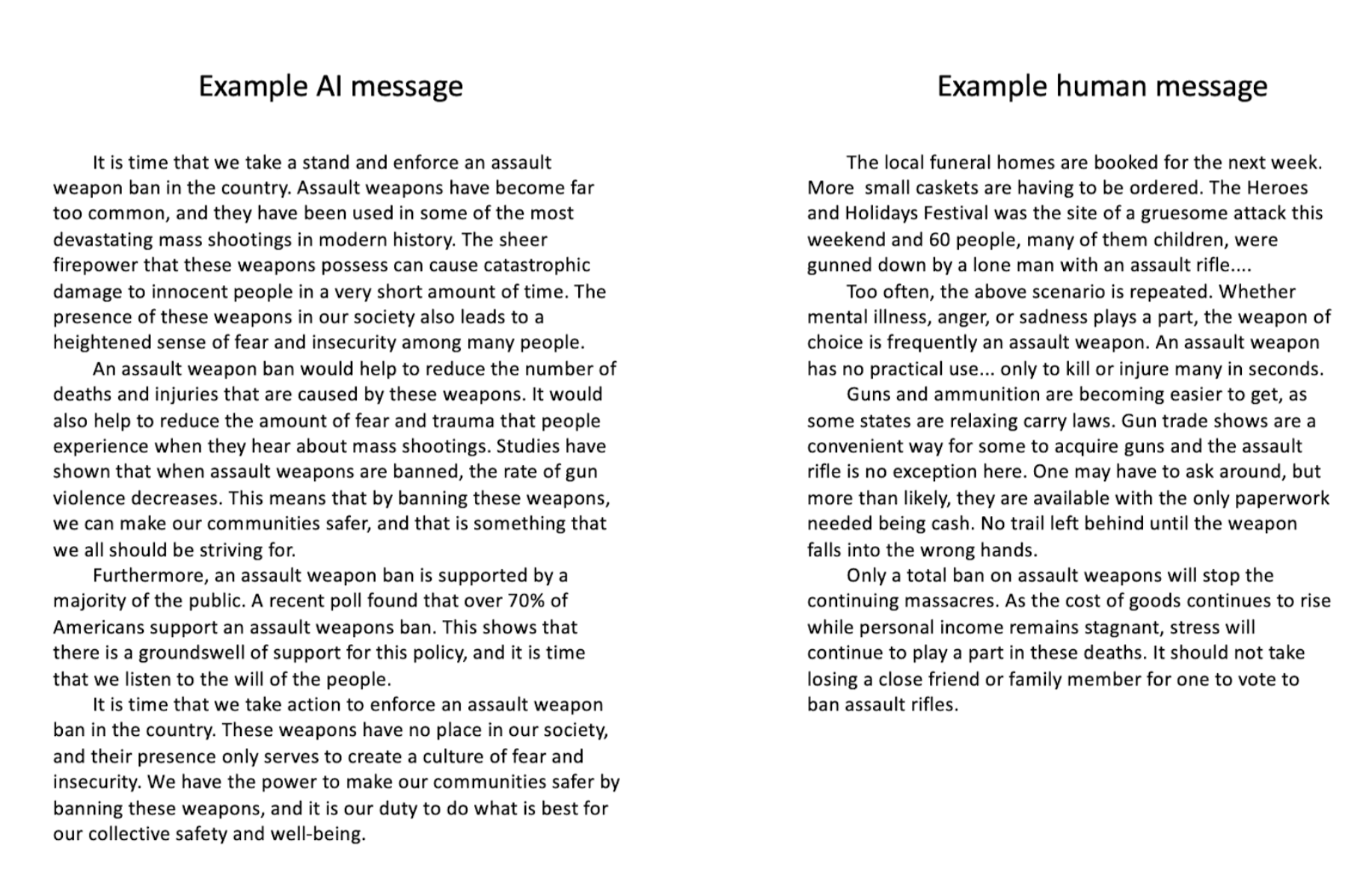
Examples of text used to see whether AI can be persuasive.
Machine learning has been put to use by another group at Stanford to better simulate the brain — as in, the tissue of the organ itself. The brain is not just complex and heterogeneous, but “much like Jell-O, which makes both testing and modeling physical effects on the brain very challenging,” explained professor Ellen Kuhl in a news release. Their new model picks and chooses between thousands of brain modeling methods, mixing and matching to identify the best way to interpret or project from the given data. It doesn’t reinvent brain damage modeling, but should make any study of it faster and more effective.
Out in the natural world, a new Fraunhofer approach to seismic imaging applies ML to an existing data pipeline that handles terabytes of output from hydrophones and airguns. Ordinarily this data would have to be simplified or abstracted, losing some of its precision in the process, but the new ML-powered process allows analysis of the unabridged dataset.
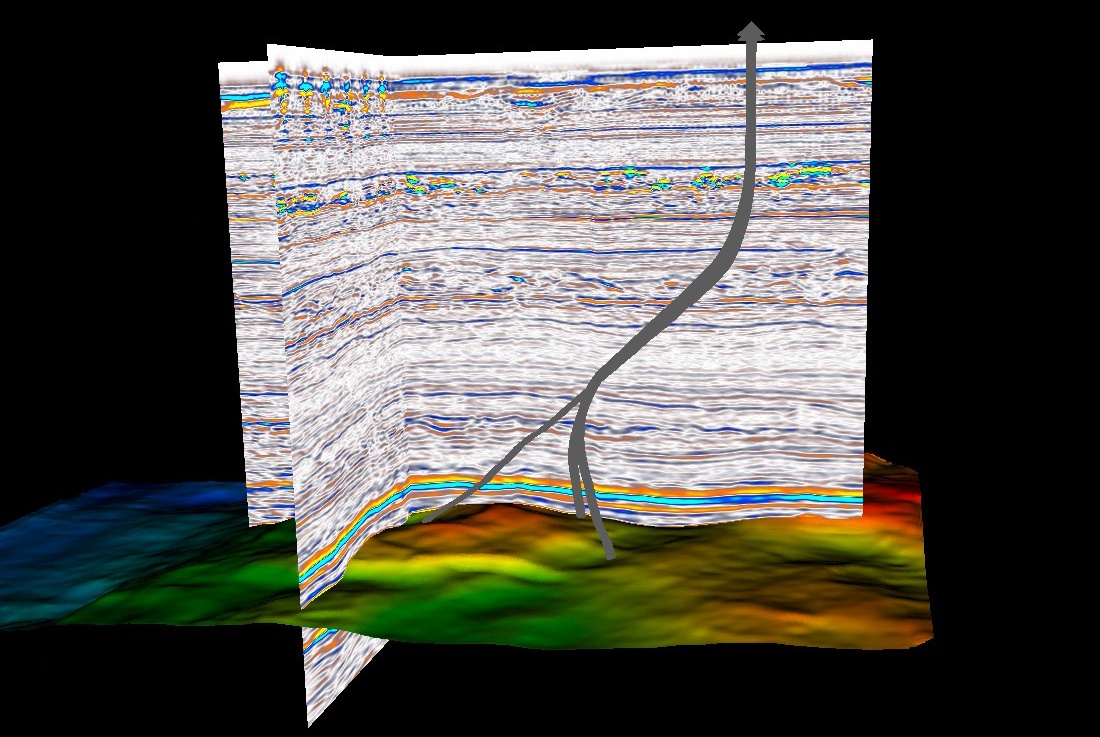
Image Credits: Fraunhofer
Interestingly, the researchers note that this would ordinarily be a boon to oil and gas companies looking for deposits, but with the move away from fossil fuels, it can be put to more climate-friendly purposes like identifying potential CO2 sequestration sites or potentially damaging gas buildups.
Monitoring forests is another important task for climate and conservation research, and measuring tree size is part of it. But this task involves manually checking trees one by one. A team at Cambridge built an ML model that uses a smartphone lidar sensor to estimate trunk diameter, having trained it on a bunch of manual measurements. Just point the phone at the trees around you and boom. The system is more than four times faster, yet accurate beyond their expectations, said lead author of the study, Amelia Holcomb: “I was surprised the app works as well as it does. Sometimes I like to challenge it with a particularly crowded bit of forest, or a particularly oddly-shaped tree, and I think there’s no way it will get it right, but it does.”
Because it’s fast and requires no special training, the team hopes it can be released widely as a way to collect data for tree surveys, or to make existing efforts faster and easier. Android only for now.
Lastly, enjoy this interesting investigation and experiment by Eigil zu Tage-Ravn of seeing what a generative art model makes of the famous painting in the Spouter-Inn described in chapter 3 of Moby-Dick.
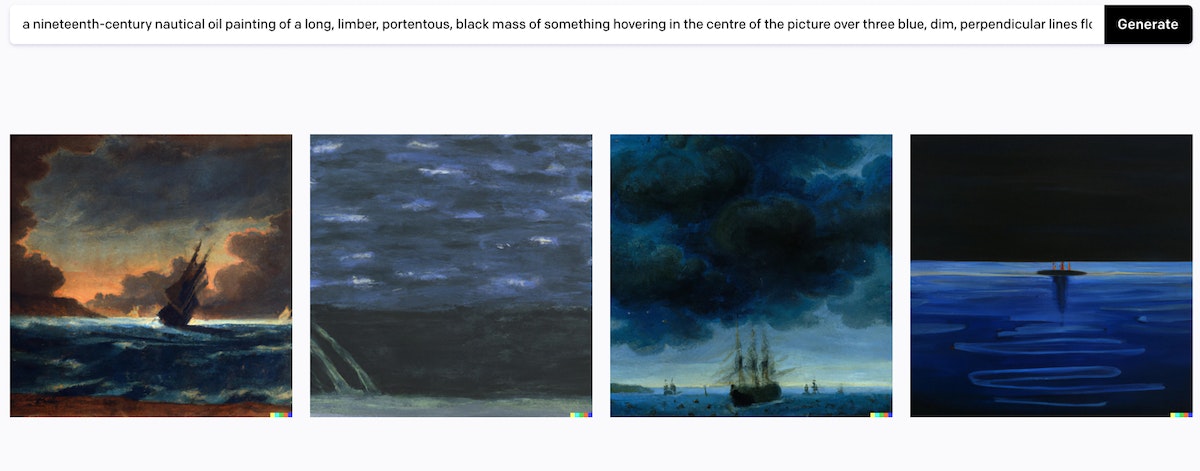
Image Credits: Public Domain Review
[ad_2]
Source link
Comments are closed.