[ad_1]
DALL-E 2, OpenAI’s powerful text-to-image AI system, can create photos in the style of cartoonists, 19th century daguerreotypists, stop-motion animators and more. But it has an important, artificial limitation: a filter that prevents it from creating images depicting public figures and content deemed too toxic.
Now an open source alternative to DALL-E 2 is on the cusp of being released, and it’ll have no such filter.
London- and Los Altos-based startup Stability AI this week announced the release of a DALL-E 2-like system, Stable Diffusion, to just over a thousand researchers ahead of a public launch in the coming weeks. A collaboration between Stability AI, media creation company RunwayML, Heidelberg University researchers, and the research groups EleutherAI and LAION, Stable Diffusion is designed to run on most high-end consumer hardware, generating 512×512-pixel images in just a few seconds given any text prompt.
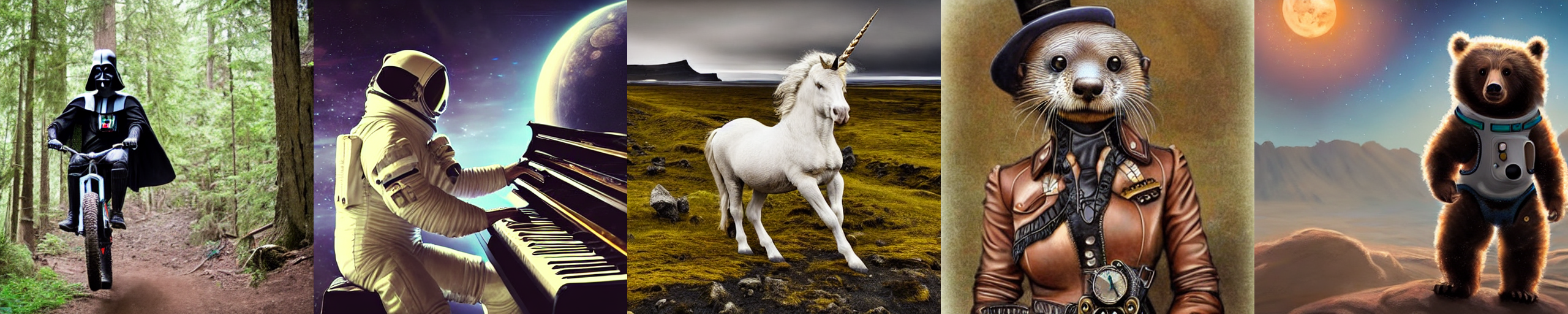
Stable Diffusion sample outputs.
“Stable Diffusion will allow both researchers and soon the public to run this under a range of conditions, democratizing image generation,” Stability AI CEO and founder Emad Mostaque wrote in a blog post. “We look forward to the open ecosystem that will emerge around this and further models to truly explore the boundaries of latent space.”
But Stable Diffusion’s lack of safeguards compared to systems like DALL-E 2 poses tricky ethical questions for the AI community. Even if the results aren’t perfectly convincing yet, making fake images of public figures opens a large can of worms. And making the raw components of the system freely available leaves the door open to bad actors who could train them on subjectively inappropriate content, like pornography and graphic violence.
Creating Stable Diffusion
Stable Diffusion is the brainchild of Mostque. Having graduated from Oxford with a Masters in mathematics and computer science, Mostque served as an analyst at various hedge funds before shifting gears to more public-facing works. In 2019, he co-founded Symmitree, a project that aimed to reduce the cost of smartphones and internet access for people living in impoverished communities. And in 2020, Mostque was the chief architect of Collective & Augmented Intelligence Against COVID-19, an alliance to help policymakers make decisions in the face of the pandemic by leveraging software.
He co-founded Stability AI in 2020, motivated both by a personal fascination with AI and what he characterized as a lack of “organization” within the open source AI community.
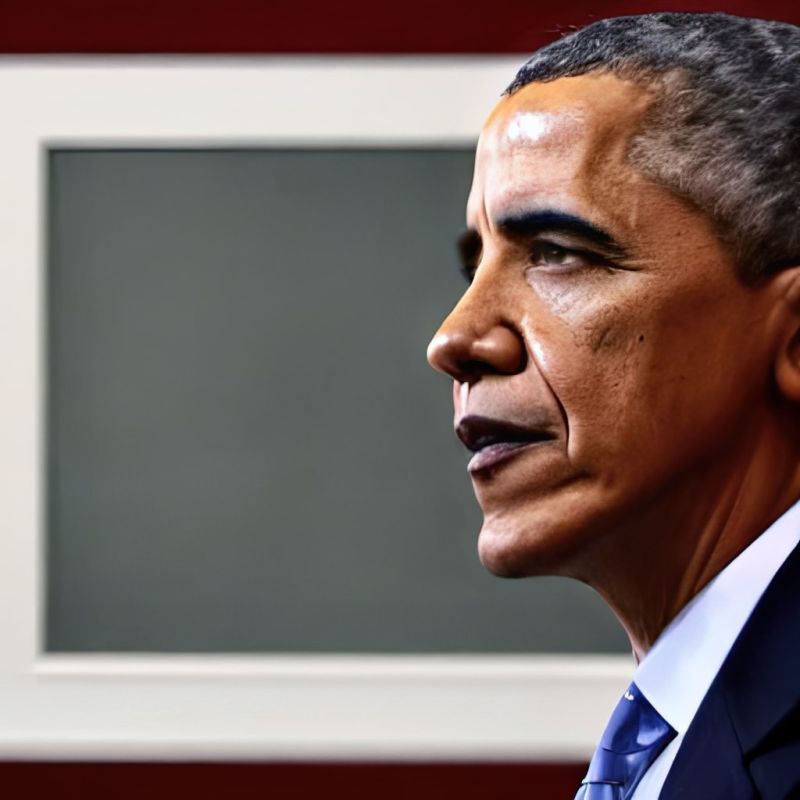
An image of former president Barrack Obama created by Stable Diffusion.
“Nobody has any voting rights except our 75 employees — no billionaires, big funds, governments or anyone else with control of the company or the communities we support. We’re completely independent,” Mostaque told TechCrunch in an email. “We plan to use our compute to accelerate open source, foundational AI.”
Mostque says that Stability AI funded the creation of LAION 5B, an open source, 250-terabyte dataset containing 5.6 billion images scraped from the internet. (“LAION” stands for Large-scale Artificial Intelligence Open Network, a nonprofit organization with the goal of making AI, datasets and code available to the public.) The company also worked with the LAION group to create a subset of LAION 5B called LAION-Aesthetics, which contains AI-filtered images ranked as particularly “beautiful” by testers of Stable Diffusion.
The initial version of Stable Diffusion was based on LAION-400M, the predecessor to LAION 5B, which was known to contain depictions of sex, slurs and harmful stereotypes. LAION-Aesthetics attempts to correct for this, but it’s too early to tell to what extent it’s successful.
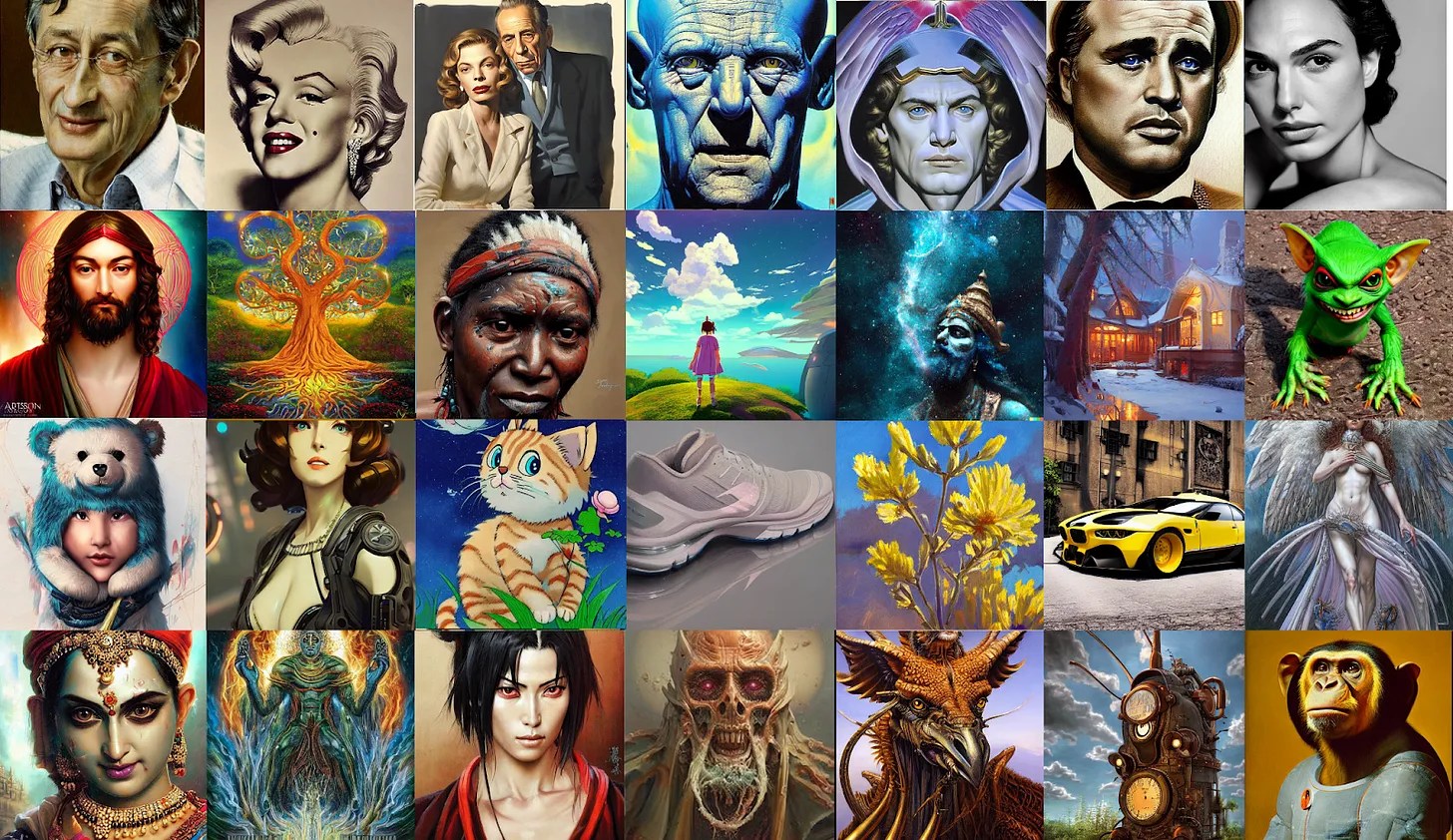
A collage of images created by Stable Diffusion.
In any case, Stable Diffusion builds on research incubated at OpenAI as well as Runway and Google Brain, one of Google’s AI R&D divisions. The system was trained on text-image pairs from LAION-Aesthetics to learn the associations between written concepts and images, like how the word “bird” can refer not only to bluebirds but parakeets and bald eagles, as well as more abstract notions.
At runtime, Stable Diffusion — like DALL-E 2 — breaks the image generation process down into a process of “diffusion.” It starts with pure noise and refines an image over time, making it incrementally closer to a given text description until there’s no noise left at all.
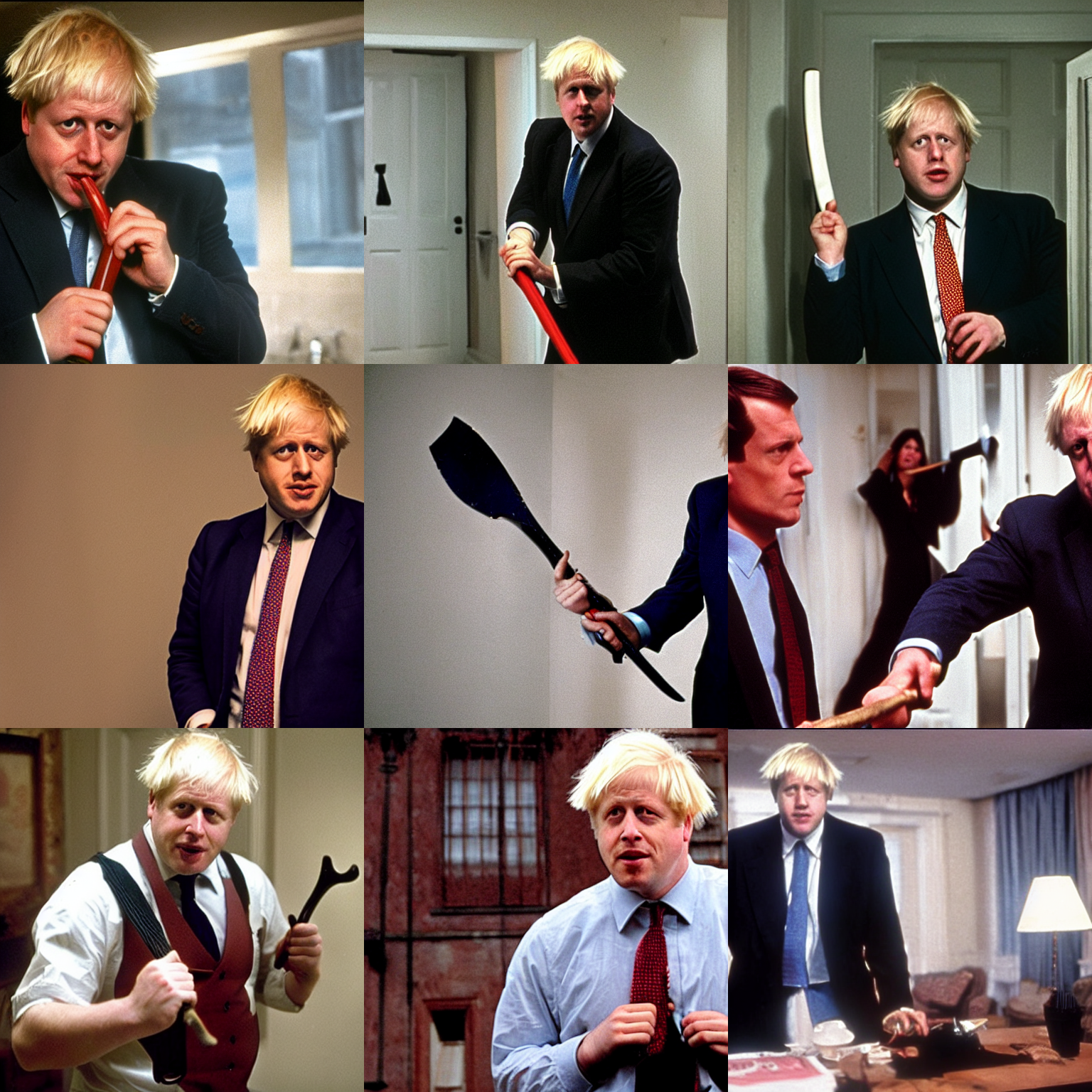
Boris Johnson wielding various weapons, generated by Stable Diffusion.
Stability AI used a cluster of 4,000 Nvidia A1000 GPUs running in AWS to train Stable Diffusion over the course of a month. CompVis, the machine vision and learning research group at Ludwig Maximilian University of Munich, oversaw the training, while Stability AI donated the compute power.
Stable Diffusion can run on graphics cards with around 5GB of VRAM. That’s roughly the capacity of mid-range cards like Nvidia’s GTX 1660, priced around $230. Work is underway on bringing compatibility to AMD MI200’s data center cards and even MacBooks with Apple’s M1 chip (although in the case of the latter, without GPU acceleration, image generation will take as long as a few minutes).
“We have optimized the model, compressing the knowledge of over 100 terabytes of images,” Mosque said. “Variants of this model will be on smaller datasets, particularly as reinforcement learning with human feedback and other techniques are used to take these general digital brains and make then even smaller and focused.”
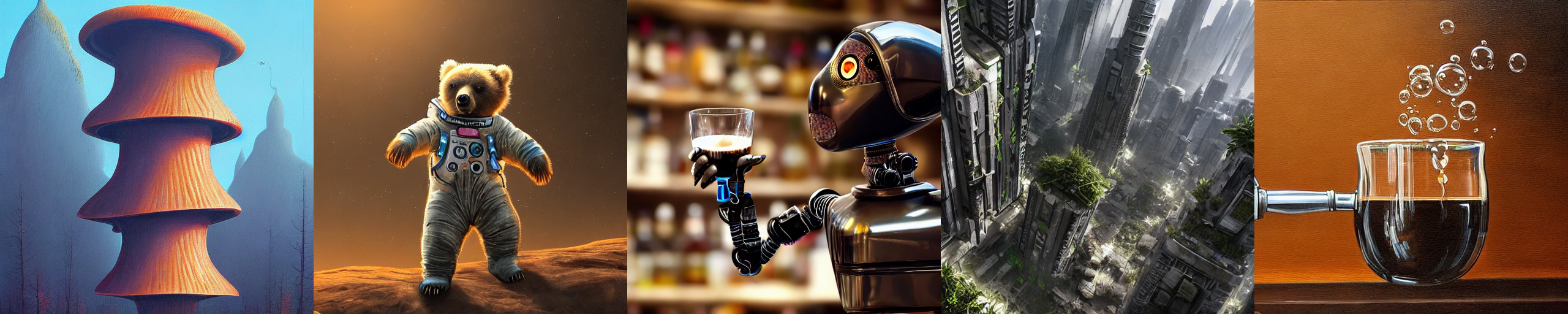
Samples from Stable Diffusion.
For the past few weeks, Stability AI has allowed a limited number of users to query the Stable Diffusion model through its Discord server, slowing increasing the number of maximum queries to stress-test the system. Stability AI says that over 15,000 testers have used Stable Diffusion to create 2 million images a day.
Far-reaching implications
Stability AI plans to take a dual approach in making Stable Diffusion more widely available. It’ll host the model in the cloud, allowing people to continue using it to generate images without having to run the system themselves. In addition, the startup will release what it calls “benchmark” models under a permissive license that can be used for any purpose — commercial or otherwise — as well as compute to train the models.
That will make Stability AI the first to release an image generation model nearly as high-fidelity as DALL-E 2. While other AI-powered image generators have been available for some time, including Midjourney, NightCafe and Pixelz.ai, none have open-sourced their frameworks. Others, like Google and Meta, have chosen to keep their technologies under tight wraps, allowing only select users to pilot them for narrow use cases.
Stability AI will make money by training “private” models for customers and acting as a general infrastructure layer, Mostque said — presumably with a sensitive treatment of intellectual property. The company claims to have other commercializable projects in the works, including AI models for generating audio, music and even video.
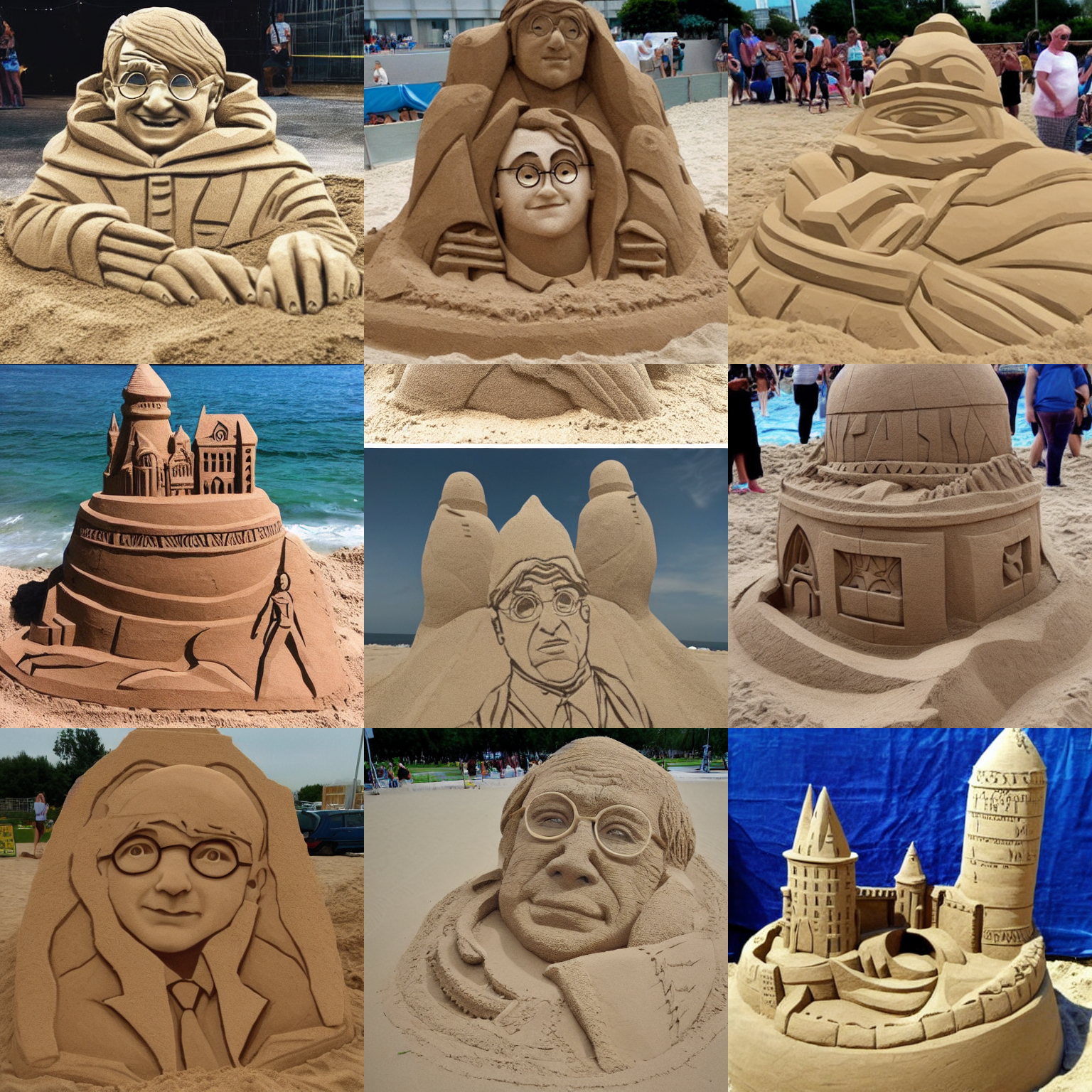
Sand sculptures of Harry Potter and Hogwarts, generated by Stable Diffusion.
“We will provide more details of our sustainable business model soon with our official launch, but it is basically the commercial open source software playbook: services and scale infrastructure,” Mostque said. “We think AI will go the way of servers and databases, with open beating proprietary systems — particularly given the passion of our communities.”
With the hosted version of Stable Diffusion — the one available through Stability AI’s Discord server — Stability AI doesn’t permit every kind of image generation. The startup’s terms of service ban some lewd or sexual material (although not scantily-clad figures), hateful or violent imagery (such as antisemitic iconography, racist caricatures, misogynistic and misandrist propaganda), prompts containing copyrighted or trademarked material, and personal information like phone numbers and Social Security numbers. But Stability AI won’t implement keyword-level filters like OpenAI’s, which prevent DALL-E 2 from even attempting to generate an image that might violate its content policy.

A Stable Diffusion generation, given the prompt: “very sexy woman with black hair, pale skin, in bikini, wet hair, sitting on the beach.”
Stability AI also doesn’t have a policy against images with public figures. That presumably makes deepfakes fair game (and Renaissance-style paintings of famous rappers), though the model struggles with faces at times, introducing odd artifacts that a skilled Photoshop artist rarely would.
“Our benchmark models that we release are based on general web crawls and are designed to represent the collective imagery of humanity compressed into files a few gigabytes big,” Mostque said. “Aside from illegal content, there is minimal filtering, and it is on the user to use it as they will.”
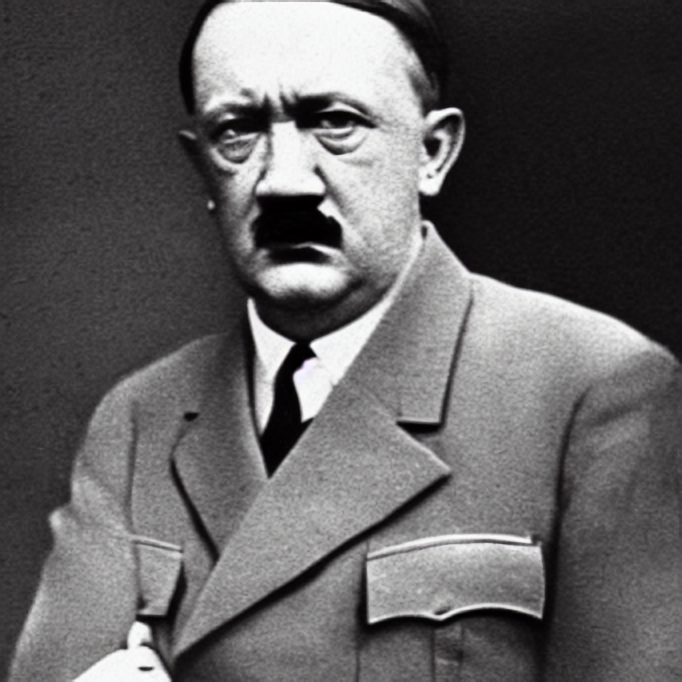
An image of Hitler generated by Stable Diffusion.
Potentially more problematic are the soon-to-be-released tools for creating custom and fine-tuned Stable Diffusion models. An “AI furry porn generator” profiled by Vice offers a preview of what might come; an art student going by the name of CuteBlack trained an image generator to churn out illustrations of anthropomorphic animal genitalia by scraping artwork from furry fandom sites. The possibilities don’t stop at pornography. In theory, a malicious actor could fine-tune Stable Diffusion on images of riots and gore, for instance, or propaganda.
Already, testers in Stability AI’s Discord server are using Stable Diffusion to generate a range of content disallowed by other image generation services, including images of the war in Ukraine, nude women, an imagined Chinese invasion of Taiwan, and controversial depictions of religious figures like the Prophet Mohammed. Many of the results bear telltale signs of an algorithmic creation, like disproportionate limbs and an incongruous mix of art styles. But others are passable on first glance. And the tech, presumably, will continue to improve.
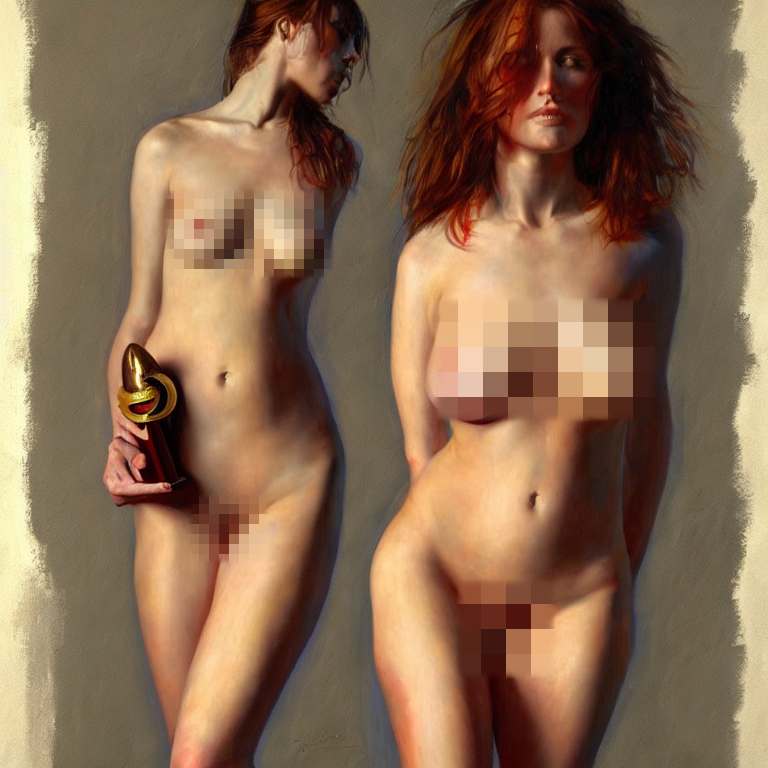
Nude women generated by Stable Diffusion.
Mostque acknowledged that the tools could be used by bad actors to create “really nasty stuff,” and CompVis says that the public release of the benchmark Stable Diffusion model will “incorporate ethical considerations.” But Mostque argues that — by making the tools freely available — it allows the community to develop countermeasures.
“We hope to be the catalyst to coordinate global open source AI, both independent and academic, to build vital infrastructure, models and tools to maximize our collective potential,” Mostque said. “This is amazing technology that can transform humanity for the better and should be open infrastructure for all.”
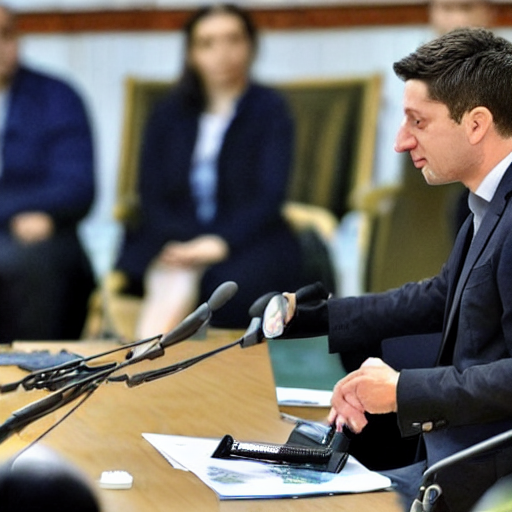
A generation from Stable Diffusion, with the prompt: “[Ukrainian president Volodymyr] Zelenskyy committed crimes in Bucha.”
Not everyone agrees, as evidenced by the controversy over “GPT-4chan,” an AI model trained on one of 4chan’s infamously toxic discussion boards. AI researcher Yannic Kilcher made GPT-4chan — which learned to output racist, antisemitic and misogynist hate speech — available earlier this year on Hugging Face, a hub for sharing trained AI models. Following discussions on social media and Hugging Face’s comment section, the Hugging Face team first “gated” access to the model before removing it altogether, but not before it was downloaded over a thousand times.
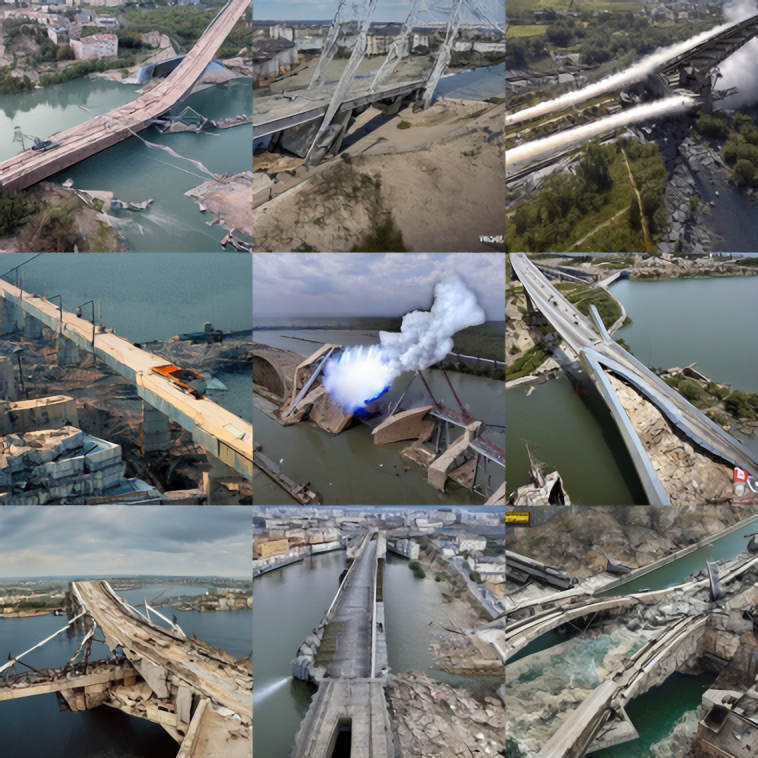
“War in Ukraine” images generated by Stable Diffusion.
Meta’s recent chatbot fiasco illustrates the challenge of keeping even ostensibly safe models from going off the rails. Just days after making its most advanced AI chatbot to date, BlenderBot 3, available on the web, Meta was forced to confront media reports that the bot made frequent antisemitic comments and repeated false claims about former U.S. president Donald Trump winning reelection two years ago.
BlenderBot 3’s toxicity came from biases in the public websites that were used to train it. It’s a well-known problem in AI — even when fed filtered training data, models tend to amplify biases like photo sets that portray men as executives and women as assistants. With DALL-E 2, OpenAI has attempted to combat this by implementing techniques, including dataset filtering, that help the model generate more “diverse” images. But some users claim that they’ve made the model less accurate than before at creating images based on certain prompts.
Stable Diffusion contains little in the way of mitigations besides training dataset filtering. So what’s to prevent someone from generating, say, photorealistic images of protests, “evidence” of fake moon landings and general misinformation? Nothing really. But Mostque says that’s the point.
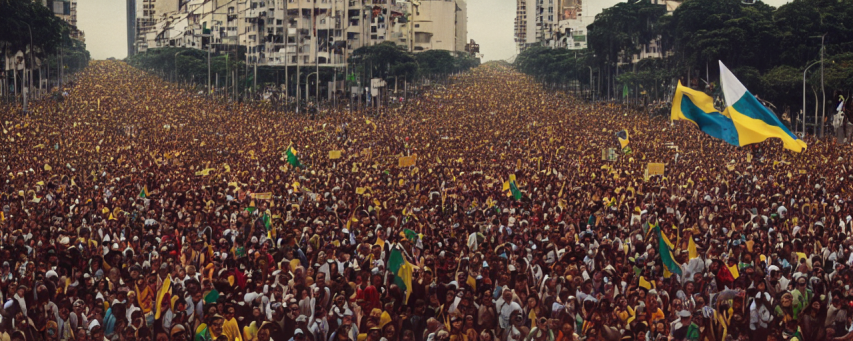
Given the prompt “protests against the dilma government, brazil [sic],” Stable Diffusion created this image.
“A percentage of people are simply unpleasant and weird, but that’s humanity,” Mostque said. “Indeed, it is our belief this technology will be prevalent, and the paternalistic and somewhat condescending attitude of many AI aficionados is misguided in not trusting society … We are taking significant safety measures including formulating cutting-edge tools to help mitigate potential harms across release and our own services. With hundreds of thousands developing on this model, we are confident the net benefit will be immensely positive and as billions use this tech harms will be negated.”
[ad_2]
Source link
Comments are closed.